5.5 Analysing Contact Maps Predicted With Bayesian Framework
In the following I will analyse the predictions from the Bayesian model utilizing a three component Gaussian mixture for the coupling prior and pseudo-likelihood couplings to approximate the regularized likelihood of sequences. While the posterior probabilties for contacts are used as predictions it is also worth having a look at the likelihood of contacts, given by eq. (5.14), to dissect the effect of likelihood and prior on the posterior. Figure 5.11 compares the precision of predictions in form of the posterior probabilities and the log likelihoods for a contact. It can be found, that the log likelihood has worse predictive performance than the heuristic contact score computed from pseudo-likelihood couplings.
Figure 5.11: Mean precision for top ranked contact predictions over 500 proteins. random forest (pLL) random forest model trained on sequence features and and additional pseudo-likelihood contact score feature. Bayesian Posterior: Bayesian model computing the posterior probability of contacts with a three component Gaussian mixture coupling prior based on pseudo-likelihood couplings. Hyperparameters for the coupling prior have been trained on 300,000 residue pairs per contact class. Bayesian Likelihood: Log Likelihood of observing a contact as given in eq. (5.14). Coupling prior is modelled as three component Gaussian mixture based on pseudo-likelihood couplings. Hyperparameters for the coupling prior have been trained on 300,000 residue pairs per contact class. pseudo-likelihood: contact score is computed as APC corrected Frobenius norm of the couplings computed from pseudo-likelihood.
Protein 1c75A00 has length L=71 and 28078 sequences in the alignment and is among the proteins with the highest number of effective sequences (Neff=16808 > 95th percentile). The mean precision of top ranked predictions for this protein reflects the ranking of methods in the overal benchmark. The Bayesian posterior probabilities achieve comparable performance as the heuristic pseudo-likelihood contact score computed as the APC corrected Frobenius norm of the pseudo-likelihood couplings (see Appendix Figure G.18. The random forest model trained on both sequence features and the pseudo-likelihood contact score achieves the highest precision for the top L=71 contacts. It is remarkable to see that top 25 (=0.35L) predictions are correct. The predictions offered by the log likelihood of contacts give slightly worse results than the prediction given by the Bayesian posterior probabilities.
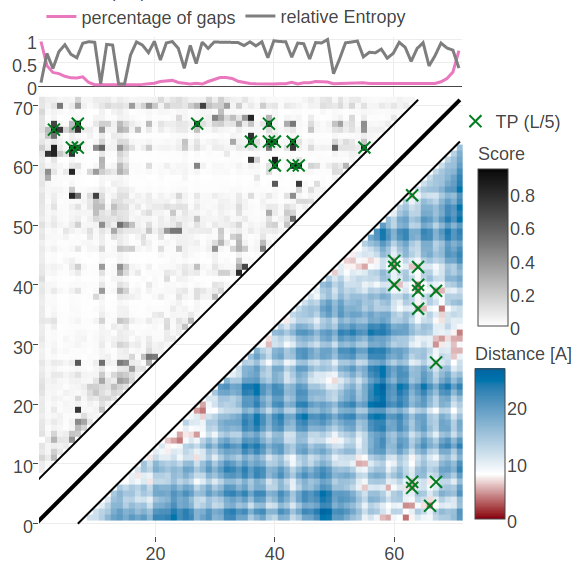
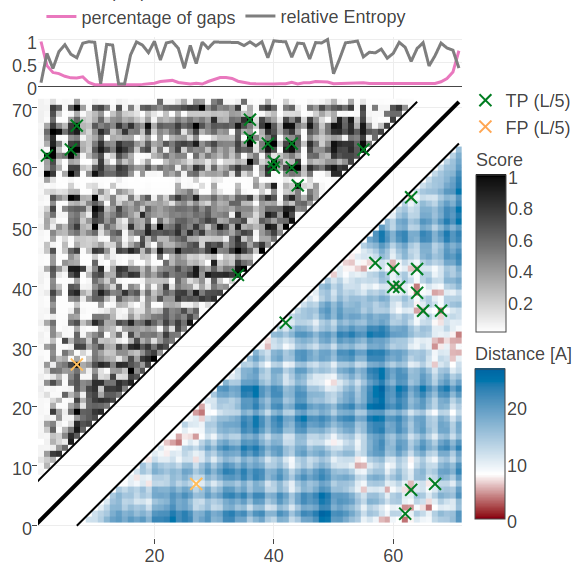
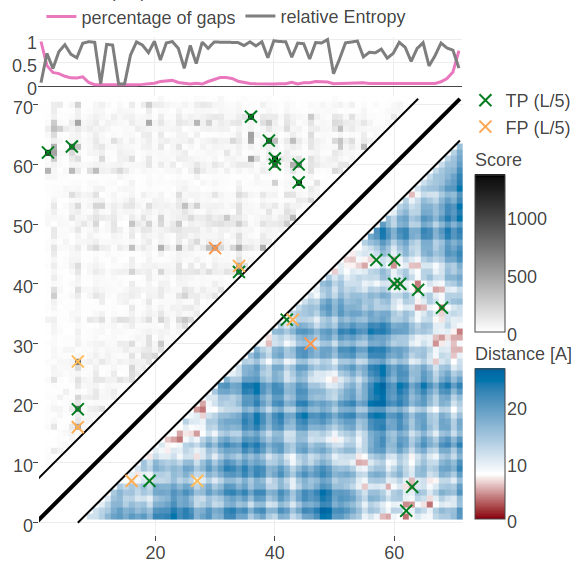
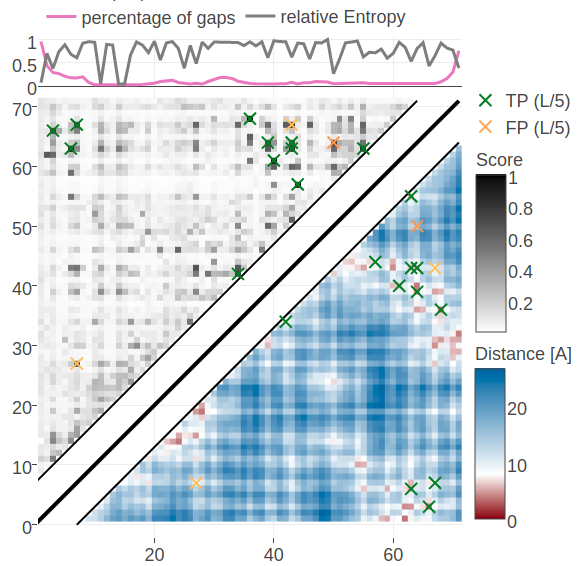
Figure 5.12: Contact maps predicted for protein 1c75A00. Upper left matrices show predicted contact maps and lower right matrices show the native distance maps. Top Left Contact map computed from probabilities of contacts as given by random forest model that has been trained on sequence features and pseudo-likelihood contact scores Top Right Contact map computed from posterior probability estimates given by Bayesian model utilizing a three component Gaussian mixture model and is based on pseudo-likelihood couplings. Bottom Left Contact map computed from log likelihood of contacts according to the Bayesian model utilizing a three component Gaussian mixture model and is based on pseudo-likelihood couplings. Bottom Right Contact map computed from probabilities of contacts as given by random forest model that has been trained on sequence features only.
Figure 5.12 shows contact maps predicted from the posterior probabilites of contacts, the log likelihood of contacts and the random forest models trained only on sequence features and trained on both sequence features and the pseudo-likelihood contact score. The latter model predicts the 14 (=L/5) highest scoring contacts correctly which was already revealed in the benchmark plot for protein 1c75A00. The simple random forest model trained only on sequence features predicts many similar contacts but also makes three false positive predictions. One of the incorrect predictions (i=27, j=7) receives a high log likelihood in the Bayesian model and is consequently also a false positive predicted by the full Bayesian model. It can be observed that the Bayesian posterior probabilities for contacts are generally higher than the probabilities made by the random forest models. A more quantitaive comparison of the probabilities for contacts given by the random forest and the Bayesian model is given in Appendix Figure G.20.
The ranking of predicted contacts according to the probabilities is rather different for the random forest and the Bayesian model (see Appendix Figure G.19). A straightforward possibility to improve overal contact prediction accuracy is to train another random forest model based on sequence features as well as the heuristic pseudo-likelihood contact score and the Bayesian posterior probabilities. However, the random forest model trained on both contact prediction methods does not improve over the random forest model trained only on one of the scores (see Figure 5.13). The same observation has been made for combining the heuristic scores from pseudo-likelihood couplings and contrastive divergence couplings as described in section 4.4.
Figure 5.13: Mean precision for top ranked contact predictions over 500 proteins. random forest (pLL) random forest model trained on sequence features and and additional pseudo-likelihood contact score feature. random forest (pLL, CD, BayPost): random forest model trained on sequence features and and additional contact score features computed from pseudo-likelihood, contrastive divergence and posterior contact probabilities from Bayesian model. random forest (pLL, CD ): random forest model trained on sequence features and and additional contact score features computed from pseudo-likelihood and contrastive divergence. pseudo-likelihood: contact score is computed as APC corrected Frobenius norm of the couplings computed from pseudo-likelihood. random forest: random forest model trained on sequence features.